Generative Models for Computer Vision
CVPR 2023, June 18th
Invited Speakers
Overview
Recent advances in generative modeling leveraging generative adversarial networks, auto-regressive models, neural fields and diffusion models have enabled the synthesis of near photorealistic images, drastically increasing the visibility and popularity of generative modeling across the computer vision research community. However, these impressive advances in generative modeling have not yet found wide adoption in computer vision for visual recognition tasks. In this workshop, we aim to bring together researchers from the fields of image synthesis and computer vision to facilitate discussions and progress at the intersection of those two subfields. We investigate the question: "How can visual recognition benefit from the advances in generative image modeling?". We invite a diverse set of experts to discuss their recent research results and future directions for generative modeling and computer vision, with a particular focus on the intersection between image synthesis and visual recognition. We hope this workshop will lay the foundation for future development of generative models for computer vision tasks.
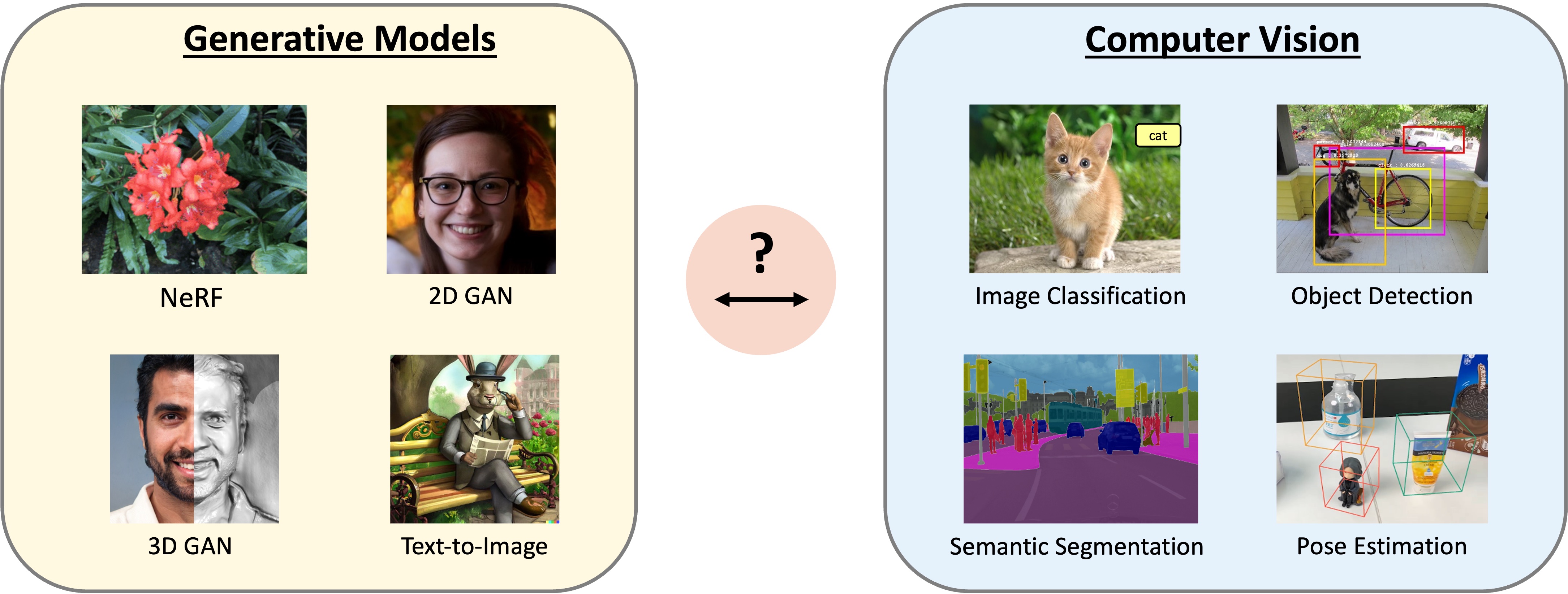
Preliminary Schedule
18th of June, UTC-7 | ||
---|---|---|
8:30 | Opening | Adam Kortylewski |
8:45 | Invited talk #1 | Alan Yuille: Analysis by Synthesis |
9:15 | Invited talk #2 | Angjoo Kanazawa: Editing 3D Scenes and Modeling 3D Social Proxemics with Generative Models |
9:45 | Invited talk #3 | Björn Ommer: Why This is (Not) the End of Research in Generative AI: Stable Diffusion & the Revolution in Visual Synthesis |
10:15 | Coffee Break | |
10:30 | Invited talk #4 | Andrea Tagliasacchi |
11:00 | Invited talk #5 | Gordon Wetzstein: A Trip Down the Generative Graphics Pipeline |
11:30 | Panel Discussion #1 | Invited Speakers #1 - #5 |
12:00 | Lunch Break | |
12:45 | Poster Session | West Building Poster 39 - 72 |
13:45 | Invited talk #6 | Angela Dai |
14:15 | Invited talk #7 | Phillip Isola: Generative Models as Data++ |
14:45 | Invited talk #8 | Vincent Sitzmann |
15:15 | Coffee Break | |
15:45 | Invited talk #9 | Christian Rupprecht: Extracting Supervision from Generative Models |
16:15 | Invited talk #10 | Shubham Tulsiani: A Probabilistic Approach to 3D Inference |
16:45 | Panel Discussion #2 | Invited Speakers #6 - #10 |
17:15 | Closing Remarks | Adam Kortylewski |
Call for Papers
-
Submission site: https://cmt3.research.microsoft.com/GCV2023/.
- Advances in generative image models
- Inversion of generative image models
- Training computer vision with realistic synthetic images
- Benchmarking computer vision with generative models
- Analysis-by-synthesis / render-and-compare approaches for visual recognition
- Self-supervised learning with generative models
- Adversarial attacks and defenses with generative models
- Out-of-distribution generalization and detection with generative models
- Ethical considerations in generative modeling, dataset and model biases
Author kit: CVPR Author KIT.
We invite submissions of both short and long papers (4 pages and 8 pages respectively excluding references). The long papers will be included in the proceedings of CVPR. Potential topics include but are not limited to:
Paper Submission Deadline
11:59 PM, March 23 (Anywhere on Earth)
Decisions
11:59 PM, April 3 (Anywhere on Earth)
Camera-Ready
11:59 PM, April 8 (Anywhere on Earth)
Accepted Papers
-
Long Papers:
-
Please refer to https://openaccess.thecvf.com/CVPR2023_workshops/GCV.
-
Text to Graphics by Program Synthesis with Error Correction [Paper]
Ivan Nikitovic (Boston University); Trisha C Anil (Boston University); Showndarya Madhavan (Boston University); Arvind Raghavan (Columbia University); Zad Chin (Harvard University); Alexander E Siemenn (Massachusetts Institute of Technology); Saisamrit Surbehera (Columbia University); Yann Hicke (Cornell University); Ed Chien (Boston University); Ori Kerret (Ven Commerce); Tonio Buonassisi (MIT); Armando Solar-Lezama (MIT); Iddo Drori (MIT)*
Short Papers:
-
Memory Efficient Diffusion Probabilistic Models via Patch-based Generation [Paper]
Shinei Arakawa (Waseda University)*; Hideki Tsunashima (National Institute of Advanced Industrial Science and Technology (AIST)); Daichi Horita (The University of Tokyo); Keitaro Tanaka (Waseda University); Shigeo Morishima (Waseda Research Institute for Science and Engineering) -
Intra-Source Style Augmentation for Improved Domain Generalization [Paper]
Yumeng Li (Bosch Center for Artificial Intelligence)*; Dan Zhang (Bosch Center for Artificial Intelligence); Margret Keuper (University of Siegen, Max Planck Institute for Informatics); Anna Khoreva (Bosch Center for Artificial Intelligence) -
A Structure-Guided Diffusion Model for Large-Hole Diverse Image Completion [Paper]
Daichi Horita (The University of Tokyo)*; Jiaolong Yang (Microsoft Research); Dong Chen (Microsoft Research Asia); Yuki Koyama (National Institute of Advanced Industrial Science and Technology (AIST)); Kiyoharu Aizawa (The University of Tokyo) -
Debiasing Scores and Prompts of 2D Diffusion for Robust Text-to-3D Generation [Paper]
Susung Hong (Korea University)*; Donghoon Ahn (Korea Unviersity); Seungryong Kim (Korea University) -
Constructive Assimilation: Boosting Contrastive Learning Performance through View Generation Strategies [Paper]
Ligong Han (Rutgers University)*; Seungwook Han (MIT-IBM Watson AI Lab, IBM Research); Shivchander Sudalairaj (MIT-IBM Watson AI Lab); Charlotte Loh (MIT); Rumen R Dangovski (MIT); Fei Deng (Rutgers University); Pulkit Agrawal (MIT); Dimitris N. Metaxas (Rutgers); Leonid Karlinsky (IBM-Research); Lily Weng (UCSD); Akash Srivastava (MIT-IBM, University Of Edinburgh) -
Self-Predicted Depth-Aware Guidance with Diffusion Models [Paper]
Gyeongnyeon Kim (Korea University )*; Wooseok Jang (Korea University); Gyuseong Lee (Korea University); Susung Hong (Korea University); Junyoung Seo (Korea University); Seungryong Kim (Korea University) -
Happy People -- Image Synthesis as Black-Box Optimization Problem in the Discrete Latent Space of Deep Generative Models [Paper]
Steffen Jung (MPII)*; Jan Christian Schwedhelm (University of Mannheim); Claudia Schillings (FU Berlin); Margret Keuper (University of Siegen, Max Planck Institute for Informatics) -
Object-Centric Slot Diffusion for Unsupervised Compositional Generation [Paper]
Jindong Jiang (Rutgers University)*; Fei Deng (Rutgers University); Gautam Singh (Rutgers University); Sungjin Ahn (KAIST) -
Visual Chain-of-Thought Diffusion Models [Paper]
William Harvey (University of British Columbia)*; Frank Wood (University of British Columbia) -
Distilling the Knowledge in Diffusion Models [Paper]
Tim Dockhorn (University of Waterloo)*; Robin Rombach (Heidelberg University); Andreas Blattmann (Heidelberg University); Yaoliang Yu (University of Waterloo) -
Scaling Robot Learning with Semantically Imagined Experience [Paper]
Tianhe Yu (Google Brain)*; Ted Xiao (Google); Austin Stone (Google); Jonathan Tompson (Google); Anthony Brohan (Google Research); Su Wang (Google AI Language); Jaspiar Singh (Google); Clayton Tan (Google); Jodilyn Peralta (Google); Brian Ichter (Google Brain); Karol Hausman (Google Brain); Fei Xia (Google Inc) -
Semi-Supervised Training of Conditional Score Models [Paper]
Chandramouli Shama Sastry (Dalhousie University/Vector Institute)*; Sri Harsha Dumpala (Dalhousie University/Vector Institute); Sageev Oore (Dalhousie University and Vector Institute) -
Multi-task View Synthesis with Neural Radiance Fields [Paper]
Shuhong Zheng (University of Illinois at Urbana-Champaign)*; Zhipeng Bao (Carnegie Mellon University); Martial Hebert (Carnegie Mellon School of Computer Science); Yu-Xiong Wang (University of Illinois at Urbana-Champaign) -
Putting People in Their Place: Affordance-Aware Human Insertion into Scenes [Paper]
Sumith Kulal (Stanford University)*; Tim Brooks (UC Berkeley); Alex Aiken (Stanford University); Jiajun Wu (Stanford University); Jimei Yang (Adobe); Jingwan Lu (Adobe Research ); Alexei A Efros (UC Berkeley); Krishna Kumar Singh (Adobe Research)
Posters:
-
Leveraging GANs for data scarcity of COVID-19: Beyond the hype [Poster]
-
DeSRF: Deformable Stylized Radiance Field [Poster]
-
Constructive Assimilation: Boosting Contrastive Learning Performance through View Generation Strategies [Poster]
-
Object-Centric Slot Diffusion for Unsupervised Compositional Generation [Poster]
-
Diversity is Definitely Needed: Improving Model-Agnostic Zero-shot Classification via Stable Diffusion [Poster]
-
Benchmarking Robustness to Text-Guided Corruptions [Poster]
-
Diffusion-Enhanced PatchMatch: A Framework for Arbitrary Style Transfer with Diffusion Models [Poster]
-
Vision + Language Applications: A Survey [Poster]
Workshop Organizers
Program Committee
Cheng Xinwen (Shanghai JiaoTong University)
Guofeng Zhang (UCLA)
Artur Jesslen (University of Freiburg)
Jiachen Sun (University of Michigan)
Jiahui Zhang (Nanyang Technological University)
Junbo Li (UC Santa Cruz)
Kibok Lee (Yonsei University)
Kunhao Liu (Nanyang Technological University)
Lifeng Huang (SunYat-sen university)
Maura Pintor (University of Cagliari)
Muyu Xu (Nanyang Technological University)
Pengliang Ji (Beihang University)
Rajkumar Theagarajan (University of California, Riverside)
Rongliang Wu (Nanyang Technological University)
Ruihao Gong (SenseTime)
Salah Ghamizi (University of Luxembourg)
Tao Li (Shanghai Jiao Tong University)
Umar Khalid (University of Central Florida)
Weijing Tao (Nanyang Technological University)
Wufei Ma (Johns Hopkins University)
Xiaoding Yuan (Johns Hopkins University)
Xingjun Ma (Deakin University)